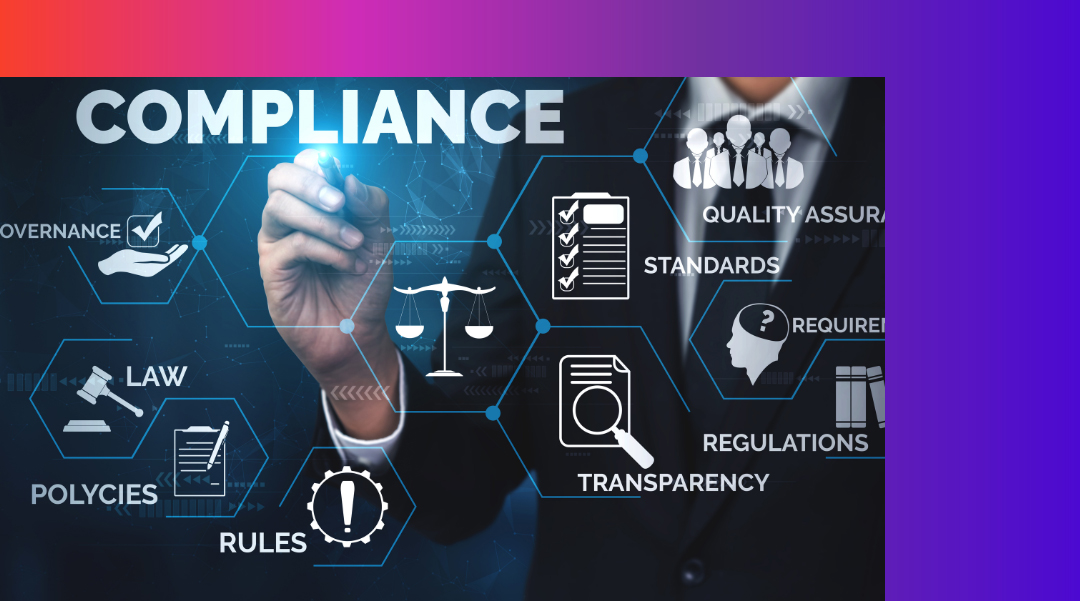
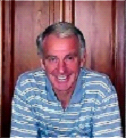
Steve Seeberg
12-2-2023
AI Recruiting Leads to Regulation and Litigation
“Black Box” Explainability Alert
Whether you are a seller of AI applications for recruiting, a customer of one of the emerging companies, or considering deploying AI for hiring in your business, this article is a must-read to avoid substantial litigation expense, potential costly penalties, and damage to your brand reputation.
While there is potential to profit from the considerable time-savings and increased productivity afforded by AI, pitfalls exist.
Artificial Intelligence (AI) is now actively being used in the recruitment process globally, streamlining hiring by analyzing millions of data points from thousands of potential job candidates in seconds.
AI typically means combining computer science and large data sets in models to enable problem-solving, make predictions, or provide recommendations. Most AI models are considered black boxes, since their decision-making processes are often opaque and difficult, if not impossible, to understand.
Application developers like Workday, Eightfold, and Beamery provide AI technology allowing their customers to scale quickly by using AI not only for matching candidates to available jobs, but also for predicting success and eliminating bias in the recruiting process.
However, a lack of transparency or “explainability” poses challenges in understanding and validating the decisions made by AI models, making it impossible to ensure lack of bias and compliance with data privacy laws in the hiring process.
I’ll provide an overview of the existing and proposed regulatory landscape as well as litigation developing to address this issue. I will also outline best practices when using AI platforms to ensure they satisfy existing and proposed regulations, as well as avoid costly lawsuits.
Given AI’s potential for injecting bias in the hiring process as well as posing threats to data security, this technology has become the new subject of large-scale regulation by the US Federal Government, US states and cities, as well as governments around the world.
More importantly, as the Workday case below shows, aggrieved applicants are not waiting for AI specific regulations to sue application providers and their customers for bias in AI algorithms. The litigant in the Workday case is testing the waters under Title VII of the Civil Rights Act of 1964 and associated legislation.
Regulation
New York City recently enacted Local Law 144 1 . The law prohibits employers from using automated employment selection tools unless an organization institutes specific bias auditing of these tools and makes the resulting data publicly available. The New York City law could be a catalyst for other states to adopt similar legislation. Liability for violations ranges from $500 to $1500 per day in addition to the potential for civil suits.
States 2 including California, Illinois, Maryland, Connecticut, Virginia, Colorado, Texas, Tennessee, Indiana, and Montana have enacted regulations covering AI learning tools as of this writing.
The US, Congress is considering the federal Algorithmic Accountability Act 3, which, if passed, would require AI vendors and employers that use their platforms to perform impact assessments of any automated decision-making systems that have a significant effect on an individuals’ access to terms, or availability of employment. The Act provides for enforcement by the FTC with the potential for significant administrative fines and exposure to civil liability.
Several states 4 , in addition to those with existing legislation noted above, have proposed regulations to address the use of AI generally which covers use in hiring.
In addition, the US Equal Employment Opportunity Commission 5 (EEOC) and National Telecommunications and Information Administration 6 (NTIA) recently announced that they intend to increase oversight and scrutiny of AI tools used to screen and hire workers
In Europe, in addition to protecting personal data privacy under General Data Protection Regulation (GDPR), the European Union recent passed draft law EU AI Act 7 with a final version expected later in the year, which aims to regulate AI hiring platforms developed or used by employers or agencies in the European Union. Proposed administrative liability is draconian, ranging from a minimum of $11 million to $33 million per violation in addition to possible civil claims by applicants. Enactment of the EU AI Act is anticipated for early 2024 and full enforcement in 2026 8.
Litigation
While AI tools for hiring have caught the attention of the EEOC, state and local legislatures, there has yet to be a proliferation of litigation in this area. However, that may soon be changing. On February 21, 2023, a class action lawsuit 9 was filed against Workday, Inc. in the Northern District Court of California, under Title VII of the Civil Rights Act of 1964 and associated statutes alleging that the company engaged in illegal race, age, and disability discrimination by offering its customers applicant screening tools that use biased AI algorithms.
It's important to note that this action was brought under existing US laws against discrimination in employment. Therefore AI vendors and their customers should consider taking the actions suggested here and recommended by most frameworks immediately, rather than wait until the implementation of regulations targeted specifically to AI tools.
Emerging Requirements
Given the current legislative proposals, it is safe to assume that both vendors and their customers may Proof via periodic internal or preferably third-party audits that AI algorithms are explainable, free of bias and user data is secure. incur liability for violations from governmental entities as well lawsuits by affected individuals. Given this likelihood, both should consider the following suggested actions when developing and/or implementing AI applications.
Although the current enacted and proposed regulations, as well as best practice recommendations, vary on approach and aspects of the hiring process affected, a common theme emerges from these and the Workday case as to actions both vendors and their customers should consider to avoid liability. These actions involve providing,
Periodic AI audits or impact assessments Proof via periodic internal or preferably third-party audits that AI algorithms are explainable, free of bias and user data is secure.
Disclosure of audit or assessment results The public is made aware of audit results on the vendors’, employers’, or agencies’ websites or by other means.
NotificationJob applicants receive information that AI is being used in the hiring process and how it affects them (“explainability”).
Key Takeaways:
Given AI’s potential for injecting bias in the hiring process as well as posing threats to data security, this technology has become the new subject of large-scale regulation by the US Federal Government, US states and cities, as well as governments around the world.
Aggrieved applicants are not waiting for AI specific regulations to sue application providers and their customers for bias in AI algorithms.
Both vendors and their customers should consider the following to avoid liability: periodic AI audits or impact assessments, disclosure of audit or assessment results, and notification of the use of AI to applicants.
Options for Application Developers & Their Customers
Depending on the regulation involved, internal AI audit and impact assessments may or may not be sufficient. It should be noted that performing these tasks internally can require significant time and use of an experienced data science team to implement the available open-source analytical tools required, depending on the use case and model complexity. However, they’re explored here as a first line of defense.
Frameworks
Frameworks are detailed guides or assessment procedures for developing and implementing AI tools that are trustworthy, satisfying the requirements of explainability, anti-bias, data security, and confidence in outcomes required by evolving regulatory schemes.
The most comprehensive framework to date is CapAI 10 , created to address the requirements of the draft EU AI Act. The Act, expected to be enacted in 2024, compliments the EU’s stringent GDPR data security regulations with respect to AI.
Additional frameworks to CapAI have been proposed from the National Institute of Standards and Technology 11 (NIST), Institute of Electrical and Electronics Engineers 12 (IEEE), Information Systems Audit and Control Association 13 (ISACA), and the Organization for Economic Co-operation and Development 14 (OECD) among others.
These frameworks, except the OECD’s, do not identify the tools required to provide audits or impact assessments required by framework protocols.
Open-source tools
Fortunately there are many open-source tools available to accomplish these tasks. However, all require programming skills and most have steep learning curves. Some of the more popular are, AI Fairness 360 15 (developed by IBM), What-If-Tool 16 (Google), Aequitas 17 , Fairlearn 18, LIME19 , Fairtest 20 , FairML 21, and more 22 .
Paid applications
Stepping in to address this problem for organizations that lack the technical capabilities or time to devote to internal AI audits or impact assessments, are third-party vendors with paid and freemium solutions.
Except for a few enterprise financial auditing and tech companies, most of these applications represent a growing cottage industry which evolved over the past AI Recruiting Leads to Regulation and Litigation 10 several years to meet anticipated demand especially from small to medium size businesses.
The largest companies offering these applications and services include; Accenture AI Services 23 (negotiated pricing), IBM Watson OpenScale 24 (“Lite” free, “Standard” $261/ model / month), PwC’s Responsible AI Toolkit 25 (negotiated pricing), Ernst & Young AI Consulting Services 26 (negotiated pricing), and KPMG Lighthouse 27 (negotiated pricing).
Smaller companies with similar offerings include; Weights & Biases 28 (freemium, from $50 per user per month), Truera 29 (negotiated pricing), Aporia 30 (freemium, quote), Fiddler 31 (free trial, quote), Arthur 32 (negotiated pricing), and Arize 33 (freemium, from $100 / mo).
Active Engagement Essential
The use of AI has resulted in a new era of efficiency in hiring, but it is not without risks. The absence of explainability and consequent lack of trust, resulting in potential bias, loss of data security and confidence in generated outcomes is worth considering if you deploy AI in hiring.
These challenges along with existing regulations, proposed legislation, and the ramifications of the Workday case demand both developers and users of this technology be more than passive observers.
They must consider actively engaging with these issues, keeping a close eye on regulatory changes, adopting appropriate frameworks, investing in the audit and impact assessment technology suggested above, as well as providing appropriate disclosure to those affected.
By taking these actions, AI vendors and their customers can leverage the substantial benefits of AI for hiring, while mitigating costly administrative penalties, potential civil liability, and reputation damage.
Notes
- https://www.nytimes.com/2023/05/25/technology/ai-hiring-law-new-york.html
- https://www.bclplaw.com/en-US/events-insights-news/2023-state-by-state-artificial-intelligence-legislation-snapshot.html
- https://www.congress.gov/bill/117th-congress/house-bill/6580/text
- https://www.bclplaw.com/en-US/events-insights-news/2023-state-by-state-artificial-intelligence-legislation-snapshot.html
- https://www.eeoc.gov/ai
- https://ntia.gov/issues/artificial-intelligence/request-for-comments
- https://artificialintelligenceact.eu/
- https://www.forbes.com/sites/forbeseq/2023/06/15/a-machine-learningengineers-guide-to-the-ai-act/?sh=24c1c41761e9
- https://storage.courtlistener.com/recap/gov.uscourts.cand.408645/gov.uscourts.cand.408645.1.0.pdf
- https://deliverypdf.ssrn.com/delivery.php?ID=79408900709101208712710 2031012103077042014005059003070089127119007022026099100085097 10703905710405603900712512601109607306600902501009005106709112 40180960681190220030140330471220940690230010060920080050860 74119064071092083078109020017031098013029098009&EXT=pdf&INDEX=TRUE
- https://nvlpubs.nist.gov/nistpubs/ai/nist.ai.100-1.pdf
- https://engagestandards.ieee.org/ieeecertifaied.html
- https://store.isaca.org/s/store#/store/browse/detail/a2S4w000004KoGpEAK
- https://www.oecd.org/science/tools-for-trustworthy-ai-008232ec-en.htm
- https://ai-fairness-360.org/
- https://pair-code.github.io/what-if-tool/
- https://github.com/dssg/aequitas
- https://github.com/fairlearn/fairlearn
- https://github.com/marcotcr/lime/
- https://github.com/columbia/fairtest
- https://github.com/adebayoj/fairml
- https://arxiv.org/pdf/2206.10613.pdf
- https://www.accenture.com/us-en/services/ai-artificial-intelligence-index
- https://cloud.ibm.com/catalog/services/watson-openscale
- https://www.pwc.com/gx/en/issues/data-and-analytics/artificial-intelligence/what-is-responsible-ai.html
- https://www.ey.com/en_us/consulting/artificial-intelligence-consulting-services
- https://advisory-marketing.us.kpmg.com/lighthouse/index.html
- https://wandb.ai/site
- https://truera.com/
- https://www.aporia.com/
- https://www.fiddler.ai/
- https://www.arthur.ai/
- https://arize.com/